Ouch! it is a lot worse than even i thought. I have been reporting on this nonsense in this blog for a long time and thought that many results were speculative but at least encouraging. Now it is anything but and yes we must utterly over haul the whole system because this happens to be awful news pretty well assuring us that the bulk of our research money is completely wasted and worse is used to produce erroneous data that others attempt to rely upon.
This also makes clear why experiments of known beneficial programs are also often in conflict.
My own experience informs me that a range of plant regimens can benefit and are normally best provided in that manner. Separating an active ingredient from a complex of synergistic ingredients strikes me as ill advised. Aspirin may well be the exception that proves the rule.
Nearly all of our medical research is wrong
So many negative results we never hear about. (Reuters/Danish Siddiqui)
Thursday, 9 June 2016
by Danielle Teller Physician and researcher
http://nexusilluminati.blogspot.ca/2016/06/nearly-all-of-our-medical-research-is.html
Something is rotten in the state of biomedical research. Everyone who works in the field knows this on some level. We applaud presentations by colleagues at conferences, hoping that they will extend the same courtesy to us, but we know in our hearts that the majority or even the vast majority of our research claims are false.
When it came to light that the biotechnology firm Amgen tried to reproduce 53 “landmark” cancer studies and managed to confirm only six, scientists were “shocked.” It was terrible news, but if we’re honest with ourselves, not entirely unexpected. The pernicious problem of irreproducible data has been discussed among scientists for decades. Bad science wastes a colossal amount of money, not only on the irreproducible studies themselves, but on misguided drug development and follow-up trials based on false information. And while unsound preclinical studies may not directly harm patients, there is an enormous opportunity cost when drug makers spend their time on wild goose chases. Discussions about irreproducibility usually ends with shrugs, however—what can we do to combat such a deep-seated, systemic problem?
Lack of reproducibility of biomedical research is not the result of an unusual level of mendacity among scientists. There are a few bad apples, but for the most part, scientists are idealistic and fervent about the pursuit of truth. The fault lies mainly with perverse incentives and lack of good management. Statisticians Stanley Young and Alan Karr aptly compare biomedical research to manufacturing before the advent of process control.
Academic medical research functions as a gargantuan cottage industry, where the government gives money to individual investigators and programs—$30 billion annually in the US alone—and then nobody checks in on the manufacturing process until the final product is delivered. The final product isn’t a widget that can be inspected, but rather a claim by investigators that they ran experiments or combed through data and made whatever observations are described in their paper. The quality inspectors, whose job it is to decide whether the claims are interesting and believable, are peers of the investigators, which means that they can be friends, strangers, competitors, or enemies.
Lack of process control leads to shoddy science in a number of ways. Many new investigators receive no standardized training. People who work in life sciences are generally not crackerjack mathematicians, and there’s no requirement to involve someone with a deep understanding of statistics. Principal investigators rarely supervise the experiments that their students and post-docs conduct alone in the lab in the dead of night, and so they have to rely on the integrity of people who are paid slave wages and whose only hope of future success is to produce the answers the boss hopes are true.
The peer review process is corrupted by cronyism and petty squabbles. These are some of the challenges inherent in a loosely organized and largely unregulated industry, but these are not the biggest reasons why so much science is unreproducible. That has more to do with dumb luck.
Randall Munroe has a wonderful cartoon at xkcd that neatly summarizes the reason why most published research findings are false. In the cartoon, scientists ask whether jelly beans cause acne and determine that they don’t. They then proceed to do subgroup analyses on 20 different colors of jelly beans, and excitedly announce that green jelly beans are associated with acne “with 95% confidence!” This is a reference to the traditional gold standard for whether or not a research finding is considered to be statistically significant. Over the last century, scientists have somewhat arbitrarily agreed that if something has only a 1-in-20 chance of happening purely by chance, then when that thing happens, we will consider it to be meaningful.
For instance, if the first time you asked someone out on a date that person declined in favor of attending a nephew’s birthday party, you might think of it as a coincidence. If the same excuse came up a second time, you might find it strange that the birthday parties always fell on Friday nights. By the third time, you would have to sadly conclude that there was a less than 1-in-20 chance that yet another nephew had a Friday night birthday party, and that the pattern of rejection was statistically significant.
One could quibble about whether or not 95% confidence is high enough to be truly confident. We wouldn’t fly on planes that had a 5% chance of crashing, but we would probably go on a picnic if there were a 5% chance of rain. Whether it’s the right number for scientific studies isn’t clear, but it is clear that this cutoff for statistical significance should not apply to multiple testing or multiple modeling.
The jelly bean cartoon illustrates this point nicely. If the scientists had found an association between jelly beans and acne on the first try, they might reasonably think that it wasn’t just chance—maybe jellybeans cause acne, or maybe acne causes jelly bean cravings. After testing 20 colors of jelly beans, though, the 1-in-20 chance of finding an association by pure chance becomes meaningless. If you test enough jelly beans, you are bound to find an association by pure chance, and that association will be spurious and irreproducible, just like many scientific studies.
When scientists run experiments in labs or model large datasets in multiple different ways, they generate heaps and heaps of negative data, but these don’t get reported. All that gets published is the 100th experiment or analysis that “worked.” Furthermore, scientists are rarely required to state upfront how they will measure primary outcomes. To understand why this is a problem, imagine that I claim to have a magic coin. I tell you that I’m going to flip it 10 times, and if it is magic, it will it come up heads every single time. That’s a pretty good study. But what if instead I flip my coin a 1,000 times and comb through the data for patterns. When I find any pattern in a series of 10 flips, and I tell you that the probability of that sequence occurring by luck alone is less than one in 1,000. That’s correct, but are you impressed by the magic of my coin?
There are some potential solutions to the irreproducibility of medical science, but they would require an extensive overhaul of the system. For observational studies, Young and Karr have proposed sensible measures, like making data publicly available, recording data analysis plans upfront, and splitting the data to be analyzed into test and validation sets. For basic science, public money could be used to set up large testing facilities where experiments can be run by impartial technicians and all results, positive or negative, can be made available to the scientific community. If such changes were implemented, however, the number of published studies would plummet precipitously. Journals would go out of business and so would most scientists, unless new criteria were devised for doling out grant money and handing out promotions.
Some areas of research would be invalidated if everyone had access to negative studies, and researchers would be discredited. The biomedical research community isn’t ready for these kinds of painful changes. One piece of evidence for this is that nobody knows which 47 studies Amgen was unable to reproduce. To gain the cooperation of the principal investigators of those studies, Amgen was forced to sign non-disclosure agreements about the results of their inquiries. It seems that the authors of the “landmark” cancer studies knew that they would be found out, and unsurprisingly, setting the record straight wasn’t high on their list of priorities.
tudy Suggests Medical Error Is Third Leading Cause of Death in US
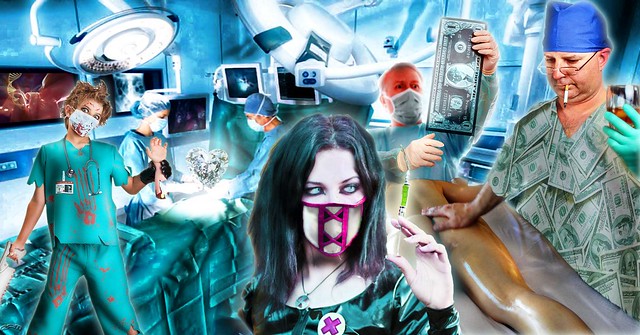
by Jackie Syrop
Medical error is the third-leading cause of death and Johns Hopkins University School of Medicine researchers are calling for better reporting on death certificates to help understand the scale of the problem and how to tackle it.
Medical error is the third-leading cause of death, after heart disease, and cancer, in the United States, according to a study published in BMJ. As a result of the findings, Johns Hopkins University School of Medicine researchers are calling for better reporting on death certificates to help understand the scale of the problem and how to tackle it.
Martin Makary, MD, MPH, professor of surgery, and Michael Daniel, a research fellow, say their research shows that US death certificates are not useful for acknowledging medical error because they rely on assigning an International Classification of Disease (ICD) code to the cause of death. If a cause of death is not associated with an ICD code, it is not captured; thus, if human and system factors are associated with a death, that is not reflected on the death certificate.
“The medical coding system was designed to maximize billing for physician services, not to collect national health statistics, as it is currently being used,” explained Makary.
Medical error is defined as an unintended act either of omission or commission or one that does not achieve its intended outcome; the failure of a planned action to be completed as intended (an error of execution); the use of a wrong plan to achieve an aim (an error of planning); or a deviation from the process of care that may or may not cause harm to the patient. This kind of error can be at the individual or system level.
Makary and Daniel used death rate data from 4 studies from 2000 to 2008, including one from the HHS’ Office of the Inspector General and the Agency for Healthcare Research and Quality. Then they used hospital admission rates from 2013 and extrapolated that based on 35,416,020 hospitalizations, there were 251,454 deaths from medical error, which translates to 9.5% of all deaths each year in the United States.
Comparing their estimate to the CDC’s list of the most common causes of death in the United States, the authors calculated that medical error is the third most common cause of death, surpassing respiratory disease—the CDC’s currently listed third leading cause of death.
Makary noted that top-ranked causes of death as reported by the CDC drive the nation’s research funding and public health priorities. While cancer and heart disease get a lot of attention, medical errors do not, and thus do not get deserved funding. More research is needed, they say, because although we cannot eliminate human error, we can better measure the problem to design safer systems mitigating its frequency, visibility, and consequences.
No comments:
Post a Comment