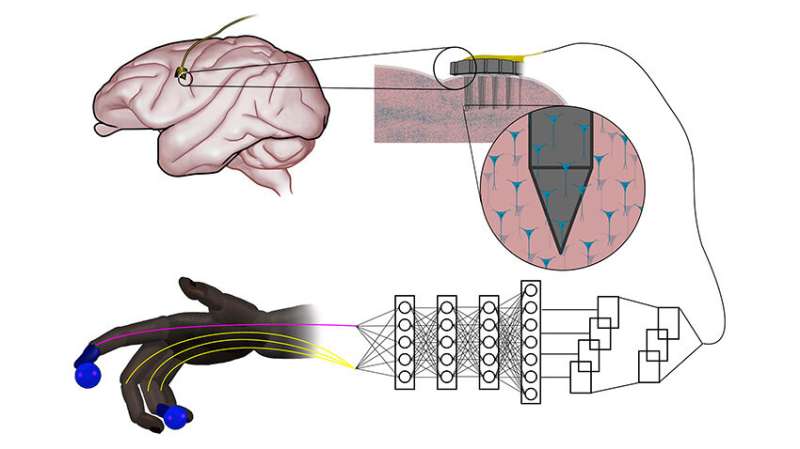
This might be huge and what catches my eye is the word simple. That is never an assumption when tackling computer supported systems but this would be wonderful
Can it really be simple? Yet we really know that our own bodies do things simply.
I do think we need to change our thinking. How about ten fingers on one hand or even having sensing attenas or even eyeballs tied to our fingers. All this opens up simple useful alternatives for operating tools.
Simple neural networks outperform more complex systems for controlling robotic prosthetics
by Jim Lynch and Kate McAlpine, University of Michigan
Intracortical arrays in motor cortex relay action potentials from biological neurons to artificial neural networks in computers that use this information to predict and control the movements of prosthetic fingers. Credit: U-M
Artificial neural networks that are inspired by natural nerve circuits in the human body give primates faster, more accurate control of brain-controlled prosthetic hands and fingers, researchers at the University of Michigan have shown. The finding could lead to more natural control over advanced prostheses for those dealing with the loss of a limb or paralysis.
The team of engineers and doctors found that a feed-forward neural network improved peak finger velocity by 45% during control of robotic fingers when compared to traditional algorithms not using neural networks. This overturned an assumption that more complex neural networks, like those used in other fields of machine learning, would be needed to achieve this level of performance improvement.
"This feed-forward network represents an older, simpler architecture—with information moving only in one direction, from input to output," said Cindy Chestek, Ph.D., an associate professor of biomedical engineering at U-M and corresponding author of the paper in Nature Communications.
"So, it was something of a surprise to us to see how it outperformed more complex systems. We feel that the feed-forward system's simplicity enables the user to have more direct and intuitive control that may be closer to how the human body operates naturally."
Fine motor skills are extremely important to humans, and the loss of this function can be devastating to people with paralysis, said first author Matthew Willsey, M.D., Ph.D., functional neurosurgery fellow at University of Michigan Health, Michigan Medicine.
RK for a random finger task. The video captures the average performance of 992 the RK as measured by throughput (1.4 bps) over the two days of testing for Monkey N when 993 comparing RK and RN. Credit: Nature Communications (2022). DOI: 10.1038/s41467-022-34452-w
"We are very motivated to use the latest techniques in machine learning to interpret neural activity from the brain for the control of dexterous finger movements," Willsey said. "We hope this line of work may help restore fine motor functioning to those who have lost it."
Advanced prosthetics and brain-computer interfaces hold the promise of returning the precise control enabled by the human hand to those with paralysis that could be caused from spinal cord injury, strokes or other injuries and diseases. But recreating the natural flow of communication between the human mind and a robotic prosthetic—with speed and precision—remains a stumbling block.
In spinal cord injury, for example, man-made neural networks can recreate the severed connection between the brain and spinal cord by using electrodes to capture impulses from the brain, interpreting them with artificial intelligence and using this to control prosthetic hands or reanimate the native limb.
But in computing, the feed-forward neural network model is thought to be less powerful for many advanced applications that use recurrent neural networks. Instead of passing input along a one-way procession, the nodes in recurrent networks have their own dynamics—the ability to create their own internal cycles via feedback, making them capable of memorizing and replaying sequences. This works extremely well when you're predicting movements from previously recorded neural data, leaving some experts to assume that this would stay the same during novel experiments.
Chestek said that, in reality, the complexity of recurrent networks for direct motor control seemed to "fight the user."
"There's nothing but a couple of neurons and a couple of synapses between the motor cortex and hand movements in the human body," she said. "There isn't a ton of processing necessary there, and the feed forward neural network may more closely resemble the natural system."
The team hopes their findings will help propel future research that can improve the speed and accuracy with which advanced prosthetics respond to the brain's impulses.
"When developing this algorithm, we tried to hold true to Einstein's well-known design principle that 'everything should be made as simple as possible, but not simpler,'" Willsey said.
"Our algorithm needs to have enough complexity to understand the possibly non-linear relationship between the electrical signals of the brain and a user's intended finger movements. However, the algorithm may one day be part of a fully implantable brain-machine interface system that restores movement to people with paralysis, and unnecessary complexity may stress these future systems in undesirable ways, such as by shortening battery life."
"At the University of Michigan, we are fortunate to have a large group of engineers, neuroscientists, and movement experts who partner together in a culture of collaboration to move the field of restorative neuroengineering forward," said Parag Patil, M.D., Ph.D., a senior author of the study and an associate professor of neurosurgery at University of Michigan Medical School. "Part of the excitement of this work is that these algorithms can be almost immediately translated to the bedside for the benefit of human research patients."
No comments:
Post a Comment