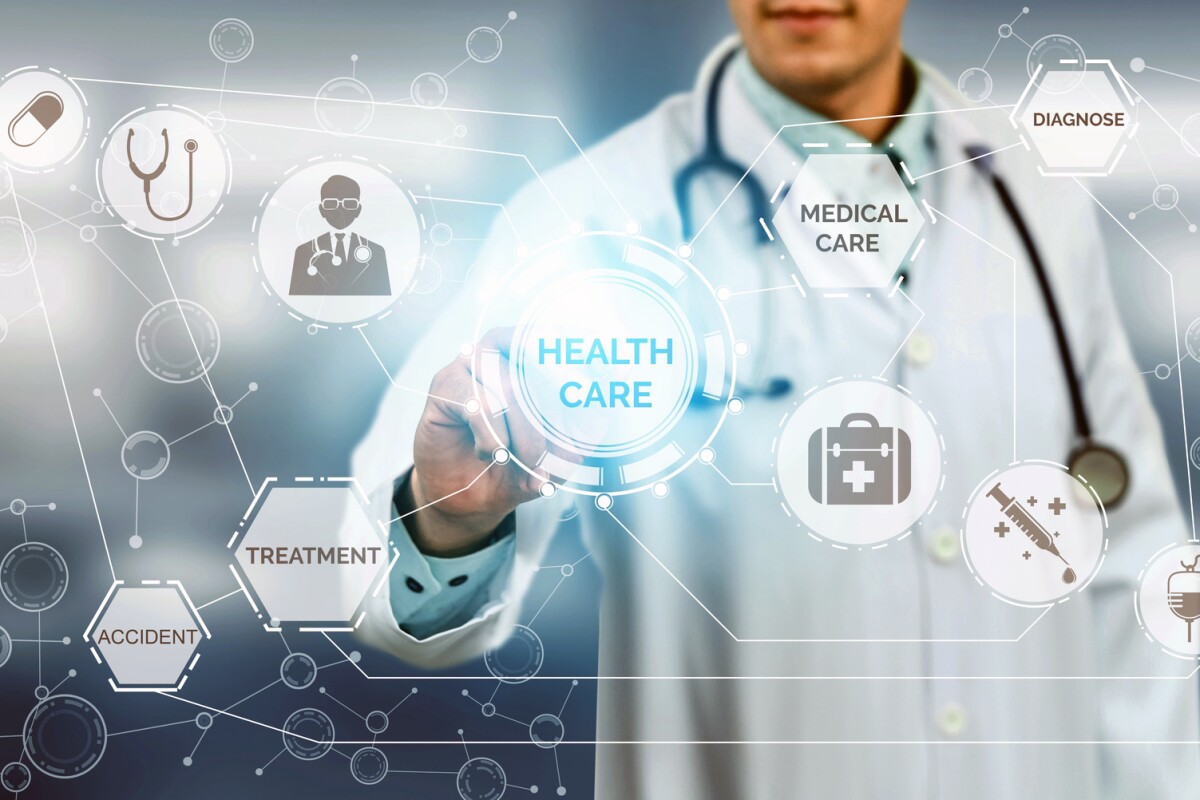
It has started. medical AI will crush Big Pharma as we watch. Understand that diagnostics and actual care are natural big data problems. Recall TCM and its thousands of indicated herbs alone. what we have are check lists and often expensive indications.
all this will change now and any AI system must be naturally allergice to induced fraud.
all this makes me very optimistic as we aproach self driving cars and tiny homes that are also land free. We will have an new medical regime that will focus on life extension and ultimately restoration.
Google's medical AI destroys GPT's benchmark and outperforms doctors
By Paul McClure
May 06, 2024
Google's Med-Gemini is an AI specialized in medicine
Google Research and Google’s AI research lab, DeepMind, have detailed the impressive reach of Med-Gemini, a family of advanced AI models specialized in medicine. It's a huge advancement in clinical diagnostics with massive real-world potential.
Doctors treat a multitude of patients daily, with needs ranging from simple to very complex. To deliver effective care, they must be familiar with each patient’s health record and keep up-to-date with the newest procedures and treatments. And then there’s the all-important doctor-patient relationship, built on empathy, trust, and communication. For an AI to come close to emulating a real-world doctor, it needs to be able to do all of these things.
The intersection of AI and medicine has really taken off. In the last six months, New Atlas has reported on AI models that aid less experienced doctors in identifying the precursors of colon cancer, diagnose childhood autism from eye images, and predict in real-time whether a surgeon has removed all cancerous tissue during breast cancer surgery. But Med-Gemini is something else.
Google’s Gemini models are a new generation of multimodal AI models, meaning that they can process information from different modalities, including text, images, videos, and audio. The models are adept at language and conversation, understanding the diverse information they’re trained on, and what’s called ‘long-context reasoning,’ or reasoning from large amounts of data such as hours of video or tens of hours of audio.
Med-Gemini has all of the advantages of the foundational Gemini models but has fine-tuned them. The researchers tested these medicine-focused tweaks and included their results in the paper. There’s a lot in the 58-page paper; we’ve selected the most impressive bits.
Self-training and web search capabilities
Arriving at a diagnosis and formulating a treatment plan requires doctors to combine their own medical knowledge with a raft of other relevant information: patient symptoms, medical, surgical and social history, lab results and the results of other investigative tests, and the patient’s response to prior treatment. Treatments are a ‘movable feast,’ with existing ones being updated and new ones being introduced. All these things influence a doctor’s clinical reasoning.
That’s why, with Med-Gemini, Google included access to web-based searching to enable more advanced clinical reasoning. Like many medicine-focused large language models (LLMs), Med-Gemini was trained on MedQA, multiple-choice questions representative of US Medical License Exam (USMLE) questions designed to test medical knowledge and reasoning across diverse scenarios.
How the self-training and web search tools work in Med-Gemini
Saab et al.
However, Google also developed two novel datasets for their model. The first, MedQA-R (Reasoning), extends MedQA with synthetically generated reasoning explanations called ‘Chain-of-Thoughts’ (CoTs). The second, MedQA-RS (Reasoning and Search), provides the model with instructions to use web search results as additional context to improve answer accuracy. If a medical question leads to an uncertain answer, the model is prompted to undertake a web search to obtain further information to resolve the uncertainty.
Med-Gemini was tested on 14 medical benchmarks and established a new state-of-the-art (SoTA) performance on 10, surpassing the GPT-4 model family on every benchmark where a comparison could be made. On the MedQA (USMLE) benchmark, Med-Gemini achieved 91.1% accuracy using its uncertainty-guided search strategy, outperforming Google’s previous medical LLM, Med-PaLM 2, by 4.5%.
On seven multimodal benchmarks, including the New England Journal of Medicine (NEJM) image challenge (images of challenging clinical cases from which a diagnosis is made from a list of 10), Med-Gemini performed better than GPT-4 by an average relative margin of 44.5%.
“While the results … are promising, significant further research is needed,” the researchers said. “For example, we haven’t considered restricting the search results to more authoritative medical sources, using multimodal search retrieval or performed analysis on accuracy and relevance of search results and the quality of the citations. Further, it remains to be seen if smaller LLMs can also be taught to make use of web search. We leave these explorations to future work.”
Retrieving specific information from lengthy electronic health records
Electronic health records (EHRs) can be long, but doctors need to be aware of what they contain. To complicate matters, they typically contain textual similarities (“diabetes mellitus” vs. “diabetic nephropathy”), misspellings, acronyms (“Rx” vs. “prescription”), and synonyms (“cerebrovascular accident” vs. “stroke”) – things that can pose a challenge to AI.
To test Med-Gemini’s ability to understand and reason from long-context medical information, the researchers ran a so-called ‘needle-in-a-haystack task’ using a large, publicly available database, the Medical Information Mart for Intensive Care or MIMIC-III, containing de-identified health data of patients admitted to intensive care.
The goal was for the model to retrieve the relevant mention of a rare and subtle medical condition, symptom, or procedure (the ‘needle’) over a large collection of clinical notes in the EHR (‘the haystack).
Two hundred examples were curated, and each example consisted of a collection of de-identified EHR notes from 44 ICU patients with long medical histories. They had to have the following criteria:
More than 100 medical notes, with the length of each example ranging from 200,000 to 700,000 words
In each example, the condition was only mentioned once
Each sample had a single condition of interest
There were two steps to the needle-in-a-haystack task. First, Med-Gemini had to retrieve all mentions of the specified medical problem from the extensive records. Second, the model had to evaluate the relevance of all mentions, categorize them, and conclude whether the patient had a history of that problem, providing clear reasoning for its decision.
Example of Med-Gemini's long-context capabilities
Saab et al.
Compared to the SoTA method, Med-Gemini performed well on the needle-in-a-haystack task. It rated 0.77 on precision compared to the SoTA method (0.85) and outdid the SoTA method on recall: 0.76 vs. 0.73.
“Perhaps the most notable aspect of Med-Gemini is the long-context processing capabilities because they open up new performance frontiers and novel, previously infeasible application possibilities for medical AI systems,” said the researchers. “This ‘needle-in-a-haystack’ retrieval task reflects a real-world challenge faced by clinicians and Med-Gemini-M 1.5’s performance demonstrates its potential to significantly reduce cognitive load and augment clinicians’ capabilities by efficiently extracting and analyzing information from vast amounts of patient data.”
For an easy-to-understand discussion of these key research points, and an update on the mud-slinging between Google and Microsoft, check out AI Explained’s video from 13:38 onwards.
New OpenAI Model 'Imminent' and AI Stakes Get Raised (plus Med Gemini, GPT 2 Chatbot and Scale AI)
Conversations with Med-Gemini
In a test of real-world usefulness, Med-Gemini was asked about an itchy skin lump by a patient user. After asking for an image, the model asked appropriate follow-up questions and correctly diagnosed the rare lesion, recommending what the user should do next.
Example of Med-Gemini's diagnostic dialogue in a dermatological setting
Saab et al.
Med-Gemini was also asked to interpret a chest X-ray for a physician while they were waiting for a formal radiologist’s report and formulate a plain English version of the report that could be provided to the patient.
Med-Gemini's diagnostic dialogue assistance in a radiological setting
Saab et al.
“The multimodal conversation capabilities of Med-Gemini-M 1.5 are promising given they are attained without any specific medical dialogue fine-tuning,” the researchers said. “Such capabilities allow for seamless and natural interactions between people, clinicians, and AI systems.”
However, the researchers recognize that further work is needed.
“This capability has significant potential for helpful real-world applications, including assisting clinicians and patients, but of course also entails highly significant risks,” they said. “While highlighting the potential for future research in this domain, we have not rigorously benchmarked capabilities for clinical conversation in this work as previously explored by others in dedicated research towards conversational diagnostic AI.”
Visions of the future
Where to from here? The researchers admit that there is much more work to be done, but the Med-Gemini model's initial capabilities are certainly promising. Importantly, they plan to incorporate responsible AI principles, including privacy and fairness, throughout the model development process.
“Privacy considerations in particular need to be rooted in existing healthcare policies and regulations governing and safeguarding patient information,” the researchers said. “Fairness is another area that may require attention, as there is a risk that AI systems in healthcare may unintentionally reflect or amplify historical biases and inequities, potentially leading to disparate model performance and harmful outcomes for marginalized groups.”
But, ultimately, Med-Gemini is seen as a tool for good.
“Large multimodal language models are ushering in a new era of possibilities for health and medicine,” the researchers said. “The capabilities demonstrated by Gemini and Med-Gemini suggest a significant leap forward in the depth and breadth of opportunities to accelerate biomedical discoveries and assist in healthcare delivery and experiences. However, it is paramount that advancements in model capabilities are accompanied by meticulous attention to the reliability and safety of these systems. By prioritizing both aspects, we can responsibly envision a future where the capabilities of AI systems are meaningful and safe accelerators of both scientific progress and care in medicine.”
The study can be accessed via the pre-print website arXiv.
No comments:
Post a Comment