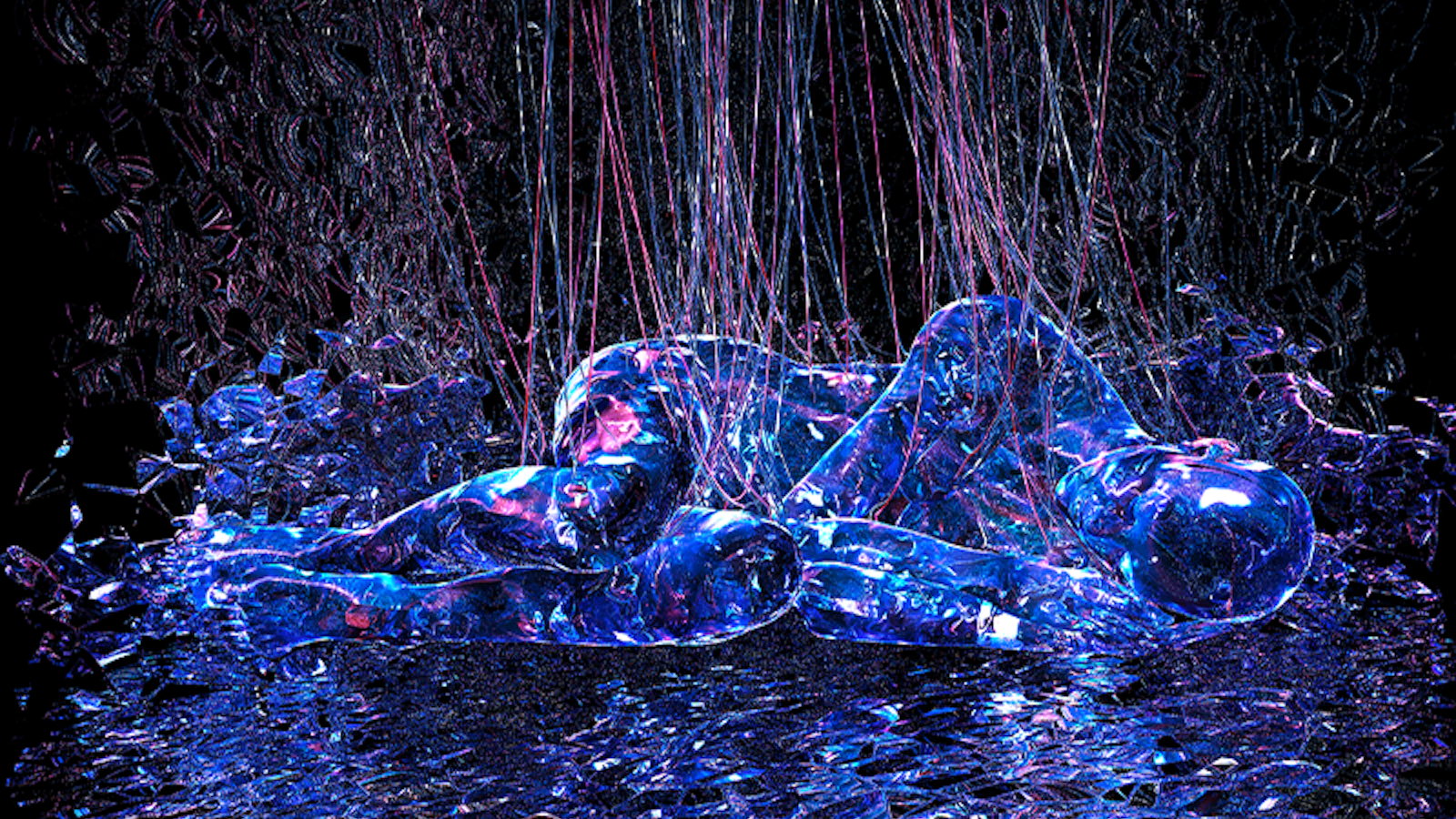
This is profound and confirms what we have suspected about sleep. I wonder if all those new imports are actually checked against future needs by actually looki0ng up future pages of TIME.
It would make great sense to do just that if that capacity is possible.
It is really handy if we can remember the future and doing so as part of memory consolidation is likely the most practical way to do it. Yet this also suggersts that real time consolidation is good enough for most day to day problems.
Even Machine Brains Need Sleep
Even Machine Brains Need Sleep
Without some downtime, artificial neural networks become catastrophically forgetful.
BY KATHERINE HARMON COURAGE
March 15, 2023
https://nautil.us/even-machine-brains-need-sleep-288191/
We all might wish for minds as retentive as a hard drive. Memory file created. Saved. Ready for access at any time. But don’t yet go wishing for the memory performance of AI.
Artificial neural networks are prone to a troublesome glitch known, evocatively, as catastrophic forgetting. These seemingly tireless networks can keep learning tasks day and night. But sometimes, once a new task is learned, any recollection of an old task vanishes. It’s as if you learned to play tennis decently well, but after being taught to play water polo, you suddenly had no recollection of how to swing a racket.
This apparent network overload put an idea in the head of Maxim Bazhenov, a professor who studies computational neuroscience and sleep at the University of California San Diego School of Medicine. Perhaps the spiking neural networks he was working with simply needed a rest.
In natural sleep, he had seen that the same basic brain processes occur in humans and in honeybees, working over information accumulated during waking moments. “That machinery presumably was doing something useful” in order to be conserved across evolutionary paths, he says. So, he thought, why not try a similar state for the machines.
The idea was to simply provide the artificial neural networks with a break from external stimuli, to instruct them to go into a sort of rest state. Like the dozing human brain, the networks were still active, but instead of taking in new information, they were mulling the old stuff, consolidating, surfacing patterns.
The networks didn’t seem to bear any resemblance to what was actually happening in a human brain.
And it worked. In a pair of papers in late 2022, Bazhenov and his colleagues showed that providing the neural networks periods in a sleep-like state mitigated the hazard of catastrophic forgetting.1, 2 It turns out that machine brains need rest, too.
The cognitive psychologist and computer scientist Geoffrey Hinton had proposed the idea of allowing early neural networks a nap in the 1990s.3 But the newer work applies the concept to radically more complex networks—in this case, what are called spiking neural networks, which mimic the pattern of neurons firing in our brains. The new work also demonstrates the use of restorative rest not only in preventing catastrophic forgetting, but also in improving generalization, both of which have implications for the true utility of these networks, from cyber security to self-driving cars (which need to remember—and sagely apply—the rules of the road, and those of Asimov).
Sleep, of course, is also essential for our own memory and learning.4 The downtime seems to strengthen new task-related connections forged in the brain during wakefulness and help transfer them to areas of the brain for longer-term storage. Researchers have known for decades that, while we might not suffer a full episode of catastrophic forgetting, lack of sleep interferes with our ability to efficiently learn new skills and retain memories.5 Newer research even suggests that we don’t need to fully power down to improve our procedural memory. Simply quietly resting while not pursuing new inputs—or as the researchers put it, engaging in “offline memory consolidation”—seems to work for human brains, too.6
Robert Stickgold raises caution here, though. Stickgold is a professor of psychiatry at Harvard Medical School’s Brain Science Initiative, where he studies sleep and cognition. Sure, it’s handy to say we’re letting a network “sleep.” But it’s wise to not take the vocabulary too far. For our sake—or for the sake of advancing network research.
Stickgold recalls a conversation he had decades ago with a researcher at MIT who was building early artificial intelligence algorithms to solve complex business problems. Stickgold commented that the networks didn’t seem to bear any resemblance to what was actually happening in a human brain. To which his engineer interlocutor replied: “Why would you want them to?” 

Katherine Harmon Courage is the deputy editor at Nautilus.
Lead image: Space Wind / Shutterstock
References
1. Golden, R., Delanois, J.E., Sanda, P., & Bazhenov, M. Sleep prevents catastrophic forgetting in spiking neural networks by forming a joint synaptic weight representation. PLOS Computational Biology 18, e1010628 (2022).
2. Tadros, T., Krishnan, G.P., Ramyaa, R., & Bazhenov, M. Sleep-like unsupervised replay reduces catastrophic forgetting in artificial neural networks. Nature Communications 13, 7742 (2022).
3. Hinton, G.E., Dayan, P., Frey, B.J., & Neal, R.M. The wake-sleep algorithm for unsupervised neural networks. Science 268, 1158-1161 (1995).
4. Walker, M.P. & Stickgold, R. Sleep-dependant learning and memory consolidation. Neuron 44, 121-133 (2004).
5. Maquet, P. The role of sleep in learning and memory. Science 294, 1048-1052 (2001).
6. Wang, S.Y., et al. “Sleep-dependant” memory consolidation? Brief periods of post-training rest and sleep provide an equivalent benefit for both declarative and procedural memory. Learning & Memory 28, 195-203 (2021).
No comments:
Post a Comment